Edge TPU inferencing overview
All inferencing with the Edge TPU is executed with TensorFlow Lite libraries. If you already have code that uses TensorFlow Lite, you can update it to run your model on the Edge TPU with only a few lines of code. We also offer Coral APIs that wrap the TensorFlow libraries to simplify your code and provide additional features.
If you're developing for a platform with a general-purpose operating system (Linux, Windows, or macOS; including a Coral Dev Board, Dev Board Mini, or Raspberry Pi), you can run an inference on the Edge TPU using either Python or C/C++ with TensorFlow Lite.
If you're developing for the Coral Dev Board Micro, then you must instead use TensorFlow Lite for Microcontrollers (TFLM), which is available in C/C++ only.
General-purpose operating systems
When you're developing for a platform that's running Linux, Windows, or macOS, you can choose to use TensorFlow Lite with either Python or C/C++.
Regardless of the language you choose, you need to install the Edge TPU runtime (libedgetpu.so), as documented in the setup for each Coral device. Then you just need the appropriate TensorFlow Lite library and optional Coral library.
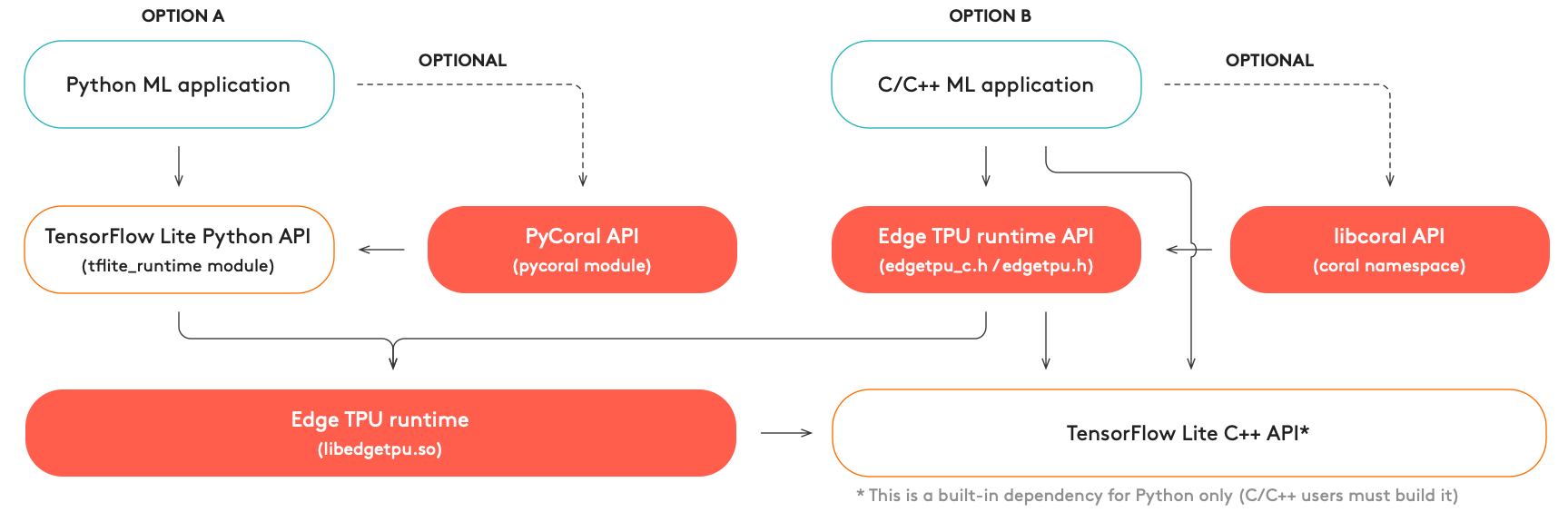
As illustrated in figure 1, you have two language options:
- For Python development:
All you need is the TensorFlow Lite Python API and the Edge TPU Runtime (libedgetpu.so).
To simplify your code, we recommend using our PyCoral API, which simplifies your code to run an inference and provides features such as pipelining a model with multiple Edge TPUs and on-device transfer learning.
To get started, read Run inference on the Edge TPU with Python.
- For C/C++ development:
All you need the Edge TPU Runtime (libedgetpu.so)—linked statically or dynamically—and the compiled TensorFlow Lite C++ library.
To simplify your code, we recommend using our libcoral API, which simplifies your code to run an inference and provides features such as pipelining a model with multiple Edge TPUs and on-device transfer learning.
For details, read Run inference on the Edge TPU with C++.
All the above software is open sourced, so you can also build these libraries for your platform.
Microcontroller systems
When you're developing for a microcontroller board such as the Coral Dev Board Micro, you must use TensorFlow Lite for Microcontrollers to execute your models, which is available only in C/C++.
You also must use certain APIs from the coralmicro library to delegate model operations to the Edge TPU, similar to the Edge TPU Runtime used with other systems above. We also recommend FreeRTOS for task scheduling and other real-time programming features.
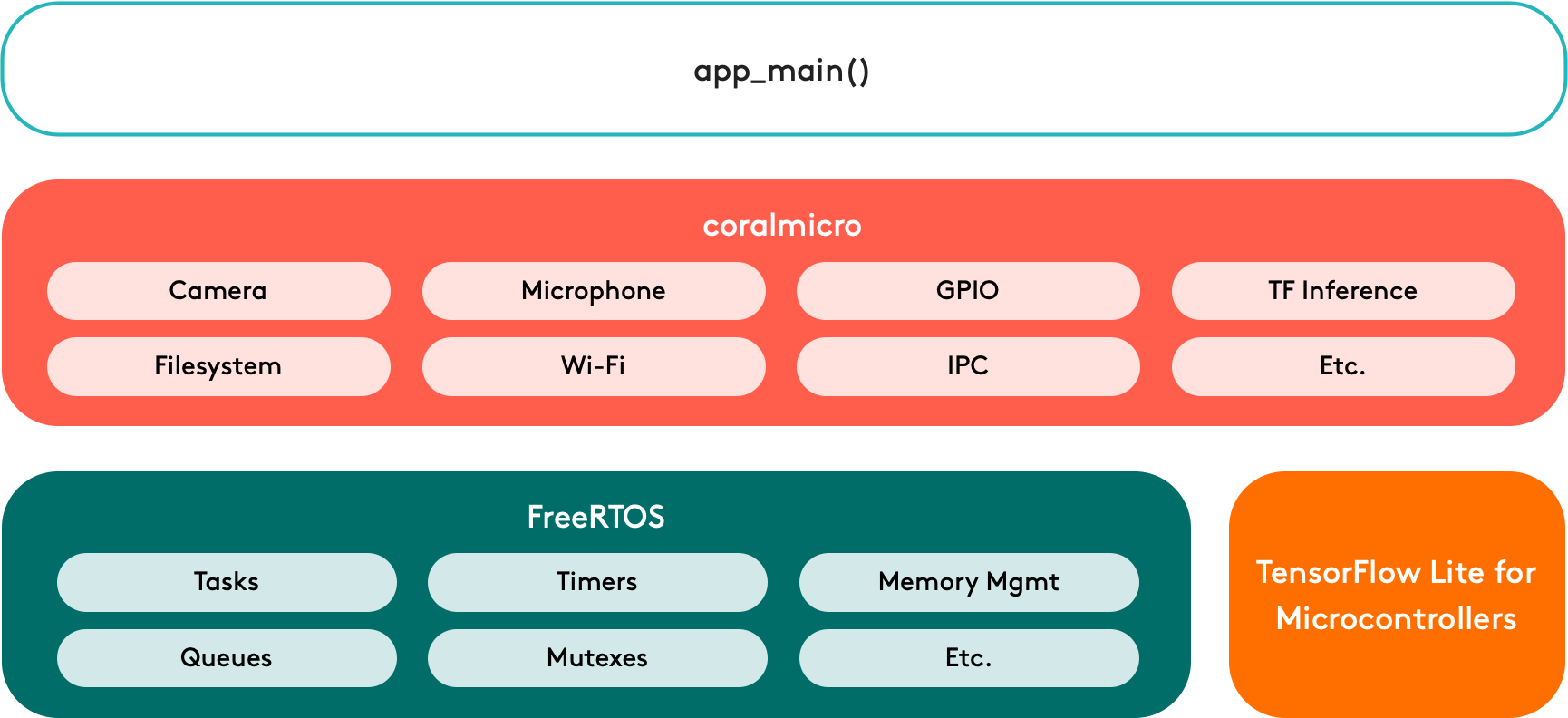
All the libraries you need to build for the Dev Board Micro are included in the coralmicro source code, including FreeRTOS and wrappers for Arduino compatibility.
To learn how to build your app for the Dev Board Micro, just follow the guide to Get started with the Dev Board Micro. Also check out the coralmicro API reference.
Is this content helpful?